GEO 580 - Lecture 3
Assessing Geographic Distributions
maps are data
maps are numbers first, pictures later
maps can be descriptive AND prescriptive
from map display to map analysis
map statistics or mapematics
Classical statistics
- central tendency (average) in numeric space
- typical measurement (average)
- how typical that typical is (std. deviation)
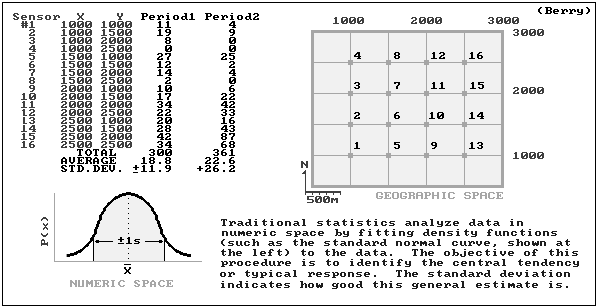
Spatial statistics
- variation (std. deviation) in geographic space
- guidance as to where the typical is too low and where it is too high
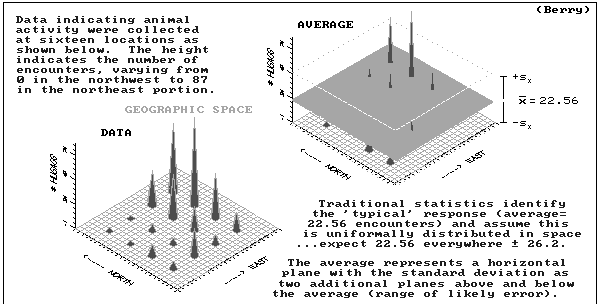
23 animals assumed everywhere?
coefficient of variation often useful
-- if std. dev. large, average is unusable
-- error flag
pitfalls of applying classical statistics to spatial data
give spatial characterization to the mean (23)
lets interpolate!
need to estimate values at locations where there are no explicit data
estimates must be determined from surrounding values
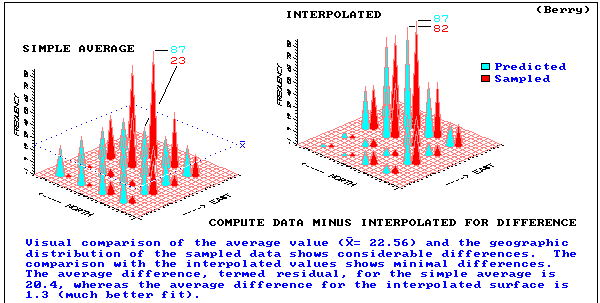
(1) FLAT plane
(2) flat but TILTED to fit data better
(3) tilted but WARPED to fit data even better
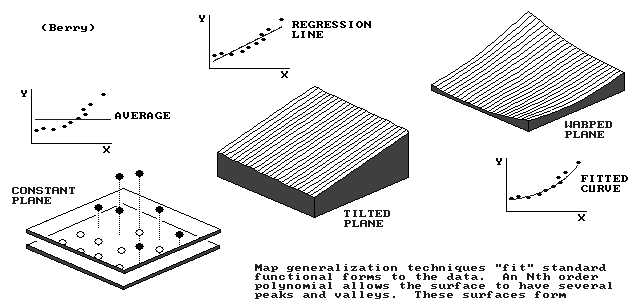
point-based
approximate interpolater
- surface doesnt pass through all data points
- global trend in data, varying slowly overlain by local but rapid fluctuations
global interpolater
- change in an input value affects the entire map
- surface is approximated by a polynomial
- output data structure is a polynomial function which can
be used to estimate values of grid points on a raster or
the value at any location
- the elevation z at any point (x,y) on the surface is
given by an equation in powers of x and y
- e.g. a linear equation (degree 1) describes a tilted
plane surface:
z = a + bx + cy
- e.g. a quadratic equation (degree 2) describes a
simple hill or valley:
z = a + bx + cy + dx² + exy + fy²
- in general, any cross-section of a surface of degree n
can have at most n-1 alternating maxima and minima
- e.g. a cubic surface can have one maximum and one
minimum in any cross-section
- equation for the cubic surface:
z = a + bx + cy + dx² + exy + fy² + gx³ + hx²y
+ ixy² + jy³
- a trend surface is a global interpolator
- assumes the general trend of the surface is
independent of random errors found at each sampled
point
- computing load is relatively light
- problems
- statistical assumptions of the model are rarely met
in practice
- edge effects may be severe
- a polynomial model produces a rounded surface
- this is rarely the case in many human and
physical applications
- available in a great many mapping packages
flat but TILTED plane to fit data
- surface is approximated by linear equation (polynomial degree 1)
z = a + bx + cy
tilted but WARPED plane to fit data
- surface is approximated by quadratic equation (polynomial degree 2)
z = a + bx + cy + dx² + exy + fy²
results extend non-spatial concept of central tendency
WHERE might you find unusual responses?
generates estimates based existing data in the region
region = roving window
- moves about study area
- summarizes data it encounters
- reach (search radius)
- number of samples
- direction
calculates an initial set of estimates at coarse gird spacing
repeatedly applies a smoothing equation (piecewise polynomial) to the surface
iterative smoothing
finer and finer grid spacings
no cliffs
no abrupt changes in slope
no kinks in contours
best for surfaces that are smooth to begin with
popular in surface interpolation packages but not common in GISs
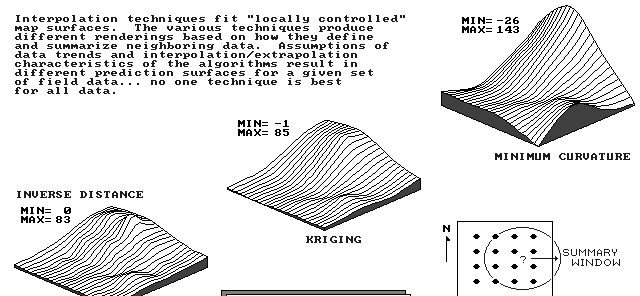
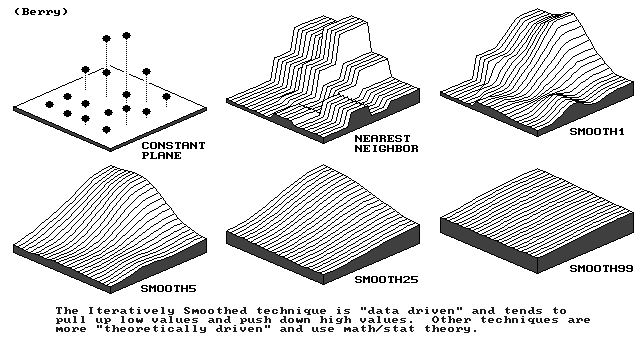
(no roving window used in nearest neighbor)
point-based
approximate interpolator
static averaging
-estimates never exceed range of data
independent random samples
- good for data with no regional trend
- developed by Georges Matheron, as the "theory of
regionalized variables", and D.G. Krige as an optimal
method of interpolation for use in the mining industry
- the basis of this technique is the rate at which the
variance between points changes over space
- this is expressed in the variogram which shows how
the average difference between values at points
changes with distance between points
Variograms
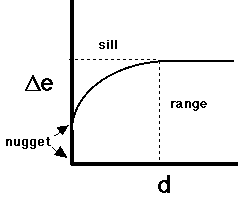
- delta-e (vertical axis) is E(zi - zj)², i.e.
"expectation" of the difference
- i.e. the average difference in elevation of any
two points distance d apart
- d (horizontal axis) is distance between i and j
- most variograms show behavior like the diagram
- the upper limit (asymptote) of delta-e is called the sill
- the distance at which this limit is reached is
called the range
- the intersection with the y axis is called the
nugget
- a non-zero nugget indicates that repeated
measurements at the same point yield different
values
- in developing the variogram it is necessary to make some
assumptions about the nature of the observed variation on
the surface:
- simple Kriging assumes that the surface has a
constant mean, no underlying trend and that all
variation is statistical
- universal Kriging assumes that there is a
deterministic trend in the surface that underlies
the statistical variation
- in either case, once trends have been accounted for (or
assumed not to exist), all other variation is assumed to
be a function of distance
Deriving the variogram
- the input data for Kriging is usually an irregularly
spaced sample of points
- to compute a variogram we need to determine how variance
increases with distance
- begin by dividing the range of distance into a set of
discrete intervals, e.g. 10 intervals between distance 0
and the maximum distance in the study area
- for every pair of points, compute distance and the
squared difference in z values
- assign each pair to one of the distance ranges, and
accumulate total variance in each range
- after every pair has been used (or a sample of pairs in a
large dataset) compute the average variance in each
distance range
- plot this value at the midpoint distance of each range
Computing the estimates
- once the variogram has been developed, it is used to
estimate distance weights for interpolation
- interpolated values are the sum of the weighted
values of some number of known points where weights
depend on the distance between the interpolated and
known points
- weights are selected so that the estimates are:
- unbiased (if used repeatedly, Kriging would give the
correct result on average)
- minimum variance (variation between repeated
estimates is minimum
- problems with this method:
- when the number of data points is large this
technique is computationally very intensive
- the estimation of the variogram is not simple, no
one technique is best
- since there are several crucial assumptions that
must be made about the statistical nature of the
variation, results from this technique can never be
absolute
- simple Kriging routines are available in the Surface II
package (Kansas Geological Survey) and Surfer (Golden
Software), and in the GEOEAS package for the PC developed
by the US Environmental Protection Agency
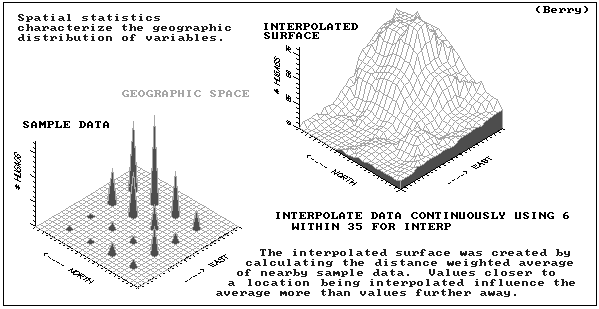
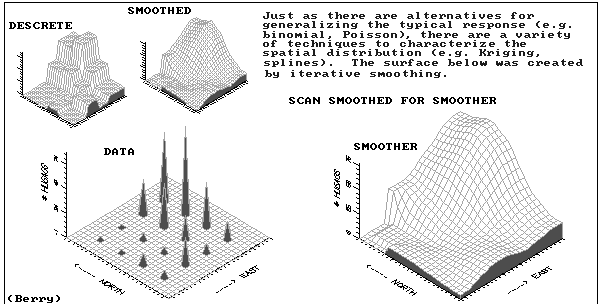
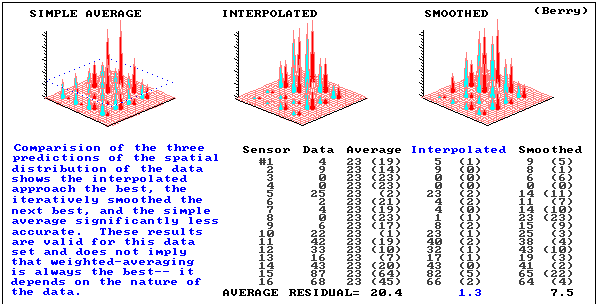
Not Discussed in Class: Fourier Series
- approximates the surface by overlaying a series of sine
and cosine waves
- a global interpolator
- computing load is moderate
- output data structure is the Fourier series which can be
used to estimate grid values for a raster or at any point
- best for data sets which exhibit marked periodicity, such
as ocean waves
- rarely incorporated in computing packages
Arc/INFO Interpolation Methods
TREND (Grid function)
SPLINE (Grid function)
IDW (Grid function)
KRIGING (Arc command)
to provide contours
to calculate some property of a surface at a given point
model all the REAL intricacies of a surface
highlight general spatial trend of data for decision-making
A GIS Perspective on Interpolation
- we've looked at point interpolation which tries to estimate a
continuous surface
- in a point case, the surface is estimated at specific
sample points
- in the case of areal interpolation, a surface
is estimated from counts within polygons (e.g.,
population density surface derived from total population
counts in each
reporting zone
- when is it impossible to conceive of a
continuous? surface??
- how about if points represent cities with attributes of
city population
- e.g., if city A has a population of 1 million and city B
100 km away has a population of 2 million, there is
no reason to believe in the existence of a city
half way between A and B with population of 1.5
million
- in this case, the variable population exists only at
the points, not as a continuous surface
- in other cases the variable might exist only along
lines
e.g. traffic density on a street network
- the above is an example of when we must distinguish between the layer and object views
of the world
- a continuous surface of elevations is a layer view
of the world - there is one value of elevation at an
infinite number of possible places in the space
- the point map of cities is an object view of the
world - the space in between points is empty, and
has no value of the population variable
- the street network is an object view of the world - the
world is empty except where there are streets - only
along streets is traffic density defined
- spatial interpolation implies a layer view of the world,
and it requires special techniques as we've discussed
to apply it to objects such as point estimates of animal
population or cities
Spatial Interpolation Algorithms in GIS
- a good GIS should include a range of spatial
interpolation routines so that the user can choose the
most appropriate method for the data and the task
- ideally, these routines should provide a natural language
interface which would lead the user through an
appropriate series of questions about the intentions,
goals and aims of the user and about the nature of the
data
- a number of prototype expert systems for guiding the
choice of a spatial interpolation algorithm have been
developed
- these may be written in the form of:
- an expert system shell (Waters, 1988)
- in one of the artificial intelligence languages such
as Prolog or LISP (see Dutton-Marion, 1988)
- or in a high level language such as Pascal (Maslyn,
1987)
- if computer contouring and surface generation techniques
are to be incorporated successfully into GIS, they must
be easy to use and effective
- "easy to use" implies that those without a detailed
knowledge of the mathematical and statistical
characteristics of the procedure should be able to
choose the correct technique for displaying a
particular data set for a particular purpose
- note: statisticians argue that this is not an
ideal goal as people may use techniques without
a proper understanding of the underlying
assumptions
- "effective" means that these techniques should be
informative, highlighting the essential nature of
the data and/or surface and serving the purpose of
the researcher/analyst
- the researcher's measure of success will be
largely subjective and visual - does the result
look right?
- the purpose of the interpolation may vary from an attempt to model all the
"real" intricacies of the surface to simply trying to
highlight the general, spatial trend of the data in order
to aid in the decision-making process
Some References
Burrough, P.A., 1986. Principles of Geographical Information
Systems for land Resources Assessment, Clarendon, Oxford.
See Chapter 8.
Lam, N., 1983. "Spatial Interpolation Methods: A Review,"
The American Cartographer 10(2):129-149.
Maslyn, R.M., 1987. "Gridding Advisor: An Expert System for
Selecting Gridding Algorithms," Geobyte 2(4):42-43.
Last updated 8 April 2000
http://dusk.geo.orst.edu/buffgis/buff03.html
Return to GEO 580 Lectures